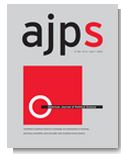
Structural topic models for open-ended survey responses
Type
Collection and especially analysis of open-ended survey responses are relatively rare in the discipline and when conducted are almost exclusively done through human coding. We present an alternative, semi-automated approach, the structural topic model (STM) (Roberts, Stewart, and Airoldi 2013; Roberts et al. 2013), that draws on recent developments in machine learning based analysis of textual data. A crucial contribution of the method is that it incorporates information about the document, such as the author’s gender, political affiliation, and treatment assignment (if an experimental study). This article focuses on how the STM is helpful for survey researchers and experimentalists. The STM makes analyzing open-ended responses easier, more revealing, and capable of being used to estimate treatment effects. We illustrate these innovations with analysis of text from surveys and experiments.
Awarded the Gosnell Prize for Excellence in Political Methodology for the best work in political methodology presented at any political science conference during the preceding year. Data at: http://dx.doi.org/10.7910/DVN/29405